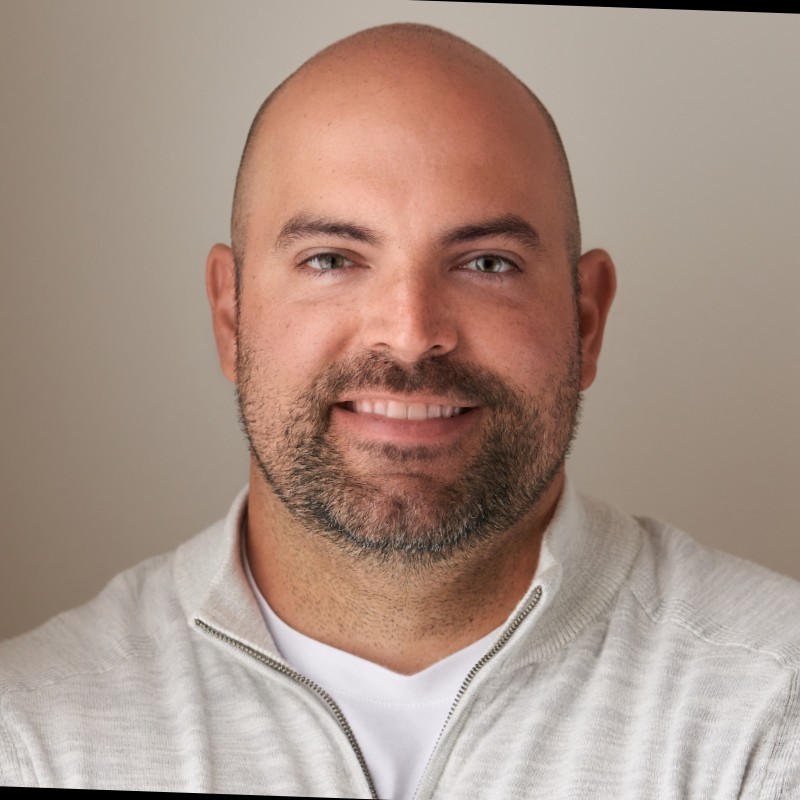
See your data in HubiFi
Just like AI has been a buzzword in all other spaces for a while, so has it in Finance and Accounting.
Everyone is asking questions about how best to apply it to their financial data. And why not? It can eliminate hours of analysis work. It can potentially uncover paths to hidden opportunities without you lifting a finger.
After all, AI can write poems, clean code, edit videos and images, and much more. Why wouldn’t it be able to dissect business models and serve up data-based recommendations on how to manage your company?
The short answer to that is that there’s more to it than it seems. These days, we’re throwing AI at everything and pretending it’ll solve all our problems. But a prerequisite to Artificial Intelligence or any sort of meaningful finance automation (and there’s a tendency to conflate the two) is data readiness.
In this post, we’ll tackle the question of how close AI is to playing a major role in your company’s finance and accounting processes.
But even more importantly, we’ll talk about data readiness. In other words, why not having centralized, unified associations of your financial data can really hurt you in the long run. Regardless of whether you intend to go down the AI path any time soon.
Delineating AI and automation has become blurry. Perhaps the best way to look at things is like this:
Underneath the surface is where most of the value is. In more understandable terms, data readiness means things like:
That's also the biggest, most demanding chunk of work. Companies today are looking at AI as a magic wand. Despite that, it’s the thing that gets you from 95% to 100% efficiency (not that that means it’s not worth investing in).
The underlying point here is that most of the power lies in how you organize your data, not technology per se.
When you become good at that, really meaningful automation and AI are the easy part, as you’re working with a great data set. You understand what operational levers drive financial performance. You begin to understand how to use AI and automation to be more efficient.
When talking about automation, it’s really a question of bifurcating between ‘dumb’ automation, like RPA, and integrated automation. With RPA, you're not doing a lot of critical thinking. You're just having a bot take over an existing process. You automate symptoms of problems, but not the actual problem. The interaction is surface-deep.
Then there's data automation or integrated automation. That's really where a subject matter expert is setting up governing how something should work end to end. That data is then ultimately automated to curate an outcome that's valuable for the business.
Rule configurations and reliable integrations become valuable tools in the hands of experts like Controllers, Financial Accountants, and Financial Systems folks. Together, they combine to enable data to be much more intentionally homogenized.
Artificial Intelligence is the next phase. AI is great for stochastic and probabilistic use cases.
You're going to be using AI to find the highest probability of converting for certain customers. Then you can double down on those investments and find these opportunities by training data against some of these models. This process is similar to how FP&A people do it. Predicting risk and predicting fraud is another great use case for AI. However, that doesn’t mean it’s omnipotent.
It's not realistic to think that an AI is going to be able to decompose your entire business model. We’re not at a point where AI can structure it into different revenue streams and deconstruct it. It can’t tell you how to optimize that based on the data it reconstructed quite yet.
For AI to really work, it takes a fair amount of data. Even high transaction businesses may not have the depth of data to be able to get the benefit that a large language model could provide.
Simply put, there’s not enough material to build these probabilistic outcomes that are more useful than an analyst doing them. And if there was, we’re probably not at a point where AI is scalable enough for smaller companies, as processing costs can skyrocket with usage.
Another aspect where AI is less applicable is areas where you need to cite sources. AI learns outputs based on hundreds of thousands and millions of transactions. So trying to cite a source back to a recommendation it made is not easy. That’s not ideal when you’re dealing with use cases like compliance.
There's a lot of things that need to happen before AI and automation can help you find value outside of individual, transaction-level optimizations and give you summarized information. In an ideal world, you would give a prompt such as:
Present X, Y, Z back to me, help me make reports or presentations for the CEO, CFO, help me take a dataset and clean it up, etc.
The application would be great for companies that have tons and tons of well-structured, associated data. Plus people from various departments trying to ask it questions. They could then benefit by efficiently and intelligently scanning that data and getting probabilistic answers on it.
But how do you get to that point?
Generative AI and productivity and analysis tools require well-defined data with clear associations. HubiFi centralizes all that data and builds clear associations based on the financial structure chart of accounts and your revenue recognition policies. You can then control that data and what you want and don’t want to share with AI.
HubiFi integrates all of your tools and financial data and creates data associations between them, including:
Whether you need a bird’s-eye view or a customer-level granular perspective, HubiFi can easily pan in or out. It provides a way to configure dynamic segmentation without compromising financial integrity.
This makes it much easier to analyze contribution margins. It helps you pinpoint under-leveraged market segments or highlight high-margin business opportunities. Insights such as these become available more quickly. That happens thanks to a built-in visualization tool based on financial statements that has immediate access to all your data, and integrates with other BI tools.
And now the infrastructure and data are there to apply finance automation and AI to your business.
On one hand, we're really overestimating the impact of AI. On the other, we’re underestimating the work we need to do from a data readiness perspective. We also underestimate the value derived from data readiness. In the next 5-10 years, there will be some serious disruption.
People who are going to win out are those who’ve invested in unifying their data in a single place that's representative of their business model. They've done the hard work and are going to reap the benefits as the power of AI becomes more widely available and scalable.
The other groups that didn't invest in that they're going to be two steps behind in five years because they will have not automated and centralized their data. They didn't have the original 0% to 80% value that they took advantage of, and they're not going to have a great way to use AI as a better advantage because they never invested in their data infrastructure in the first place.
According to McKinsey, data-driven companies are 23 times more likely to top their competitors in customer acquisition. They are also about 19 times more likely to stay profitable and nearly seven times more likely to retain customers. HubiFi is positioned perfectly for you to make this data-first pivot. It will gradually open the door to incremental benefits of AI.
You will gain more efficiencies and glean more insights, but the big first step in that investment is centralizing and automating all that data so that your team moves from transactional to strategic analysis.
After that, the strategic analysis is going to get more and more valuable over time. As you expose and learn language models, you will be able to identify opportunities more quickly. And then that happens more profitably with less effort from human beings.
In general, data readiness can be broken down into 5 high-level steps:
Over the next five years, the companies that invested in data readiness will reap the benefits of automation and AI. Those who haven’t will scramble to adopt AI, however without the necessary prerequisite.
Other than a few individual tasks, that won’t lead to a whole lot of optimization and time savings. That’s because for AI and automation to have a holistic effect on your company, finance data from operational sources needs to be interconnected. It needs structure and data readiness.
And HubiFi has positioned itself perfectly to help centralize your data and do the hardest part of the job for you. Take the first step to introducing automation and AI to your business and schedule a free conversation with the founder of HubiFi here.
Former Root, EVP of Finance/Data at multiple FinTech startups
Jason Kyle Berwanger: An accomplished two-time entrepreneur, polyglot in finance, data & tech with 15 years of expertise. Builder, practitioner, leader—pioneering multiple ERP implementations and data solutions. Catalyst behind a 6% gross margin improvement with a sub-90-day IPO at Root insurance, powered by his vision & platform. Having held virtually every role from accountant to finance systems to finance exec, he brings a rare and noteworthy perspective in rethinking the finance tooling landscape.